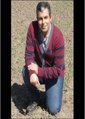
Mohsen Mohammadi
Purdue University, USA
Title: PopVar: Plant breeder’s tool to predict genetic variance and trait correlations in bi-parental crosses
Biography
Biography: Mohsen Mohammadi
Abstract
Improvement of quantitative traits depends highly on the right choice of parent combinations. The ability to predict parent combinations that produce high progeny means and high progeny variances improves the effectiveness of breeding cycles. PopVar is a tool that predicts population mean, genetic variance and the mean of superior progeny per cross single trait and also correlated responses for multiple traits. PopVar uses Stahl’s model of recombination to produce in silico recombinant inbred lines (RILs) per each cross using the genotype information of the respective parental lines. Then, a genome-wide prediction model, trained on a set of relevant germplasm and traits of interest is used to predict the breeding values of each simulated RIL within a cross. The predicted breeding values per cross are then used to calculate genetic parameters such as mean, variance and the mean of the superior tail of the population for each trait and co variations of trait pairs. We used a training population composed of 383 breeding lines, genotyped with 1142 SNP markers and phenotyped for yield and deoxynivalenol (DON) to demonstrate the utility of the PopVar procedure for each trait individually and for co variation of the two traits together. Our data established that population mean (mid-parent value) alone explained 82 and 88% of variation of the superior progeny mean for yield and DON, respectively and adding predicted population variance to the regression model increased R2 values to 99.5 and 99.6%, respectively. The results of correlated response revealed that although yield and DON are unfavorably correlated, the correlation was near zero or slightly negative in some simulated crosses, indicating the potential to increase yield while decreasing DON. Historical methods of parent selection strategies do not provide direct measures of variance. Rather, they either use genotype information irrespective of phenotype (genetic distance) or they use phenotypic distances irrespective of genotype information (phenotypic distance). In contrast, PopVar explicitly models segregation of the underlying genetic effects for a trait within a population. This work extends the current benefits of genomic selection to include the ability to design crosses that maximize genetic variance with more favorable correlations among traits. PopVar is available as an R package that researchers and breeders are encouraged to use for empirical evaluation of the methodology.